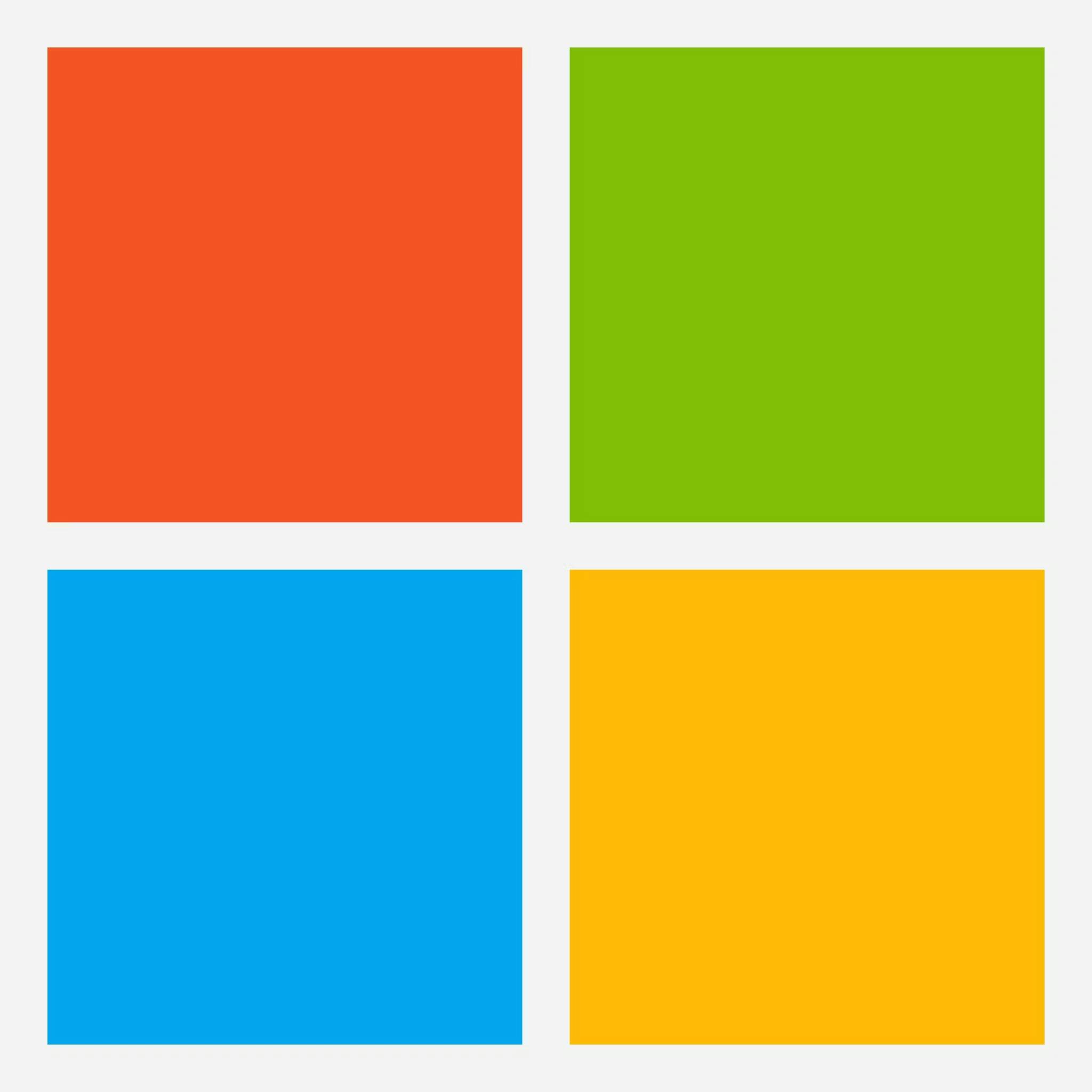
Paradigm / Shift: Stories of innovation, shaped by intelligence.
Listen to an audio version of this story, narrated by Harsha Bhogle.
Editor’s note: This story is a textual version of the podcast’s script. It has been edited and condensed for clarity. This story and the podcast are brought to you in partnership with Microsoft India.
On 29 October 1999, a “Super Cyclone” called Paradip devastated parts of Odisha and the east coast of India. At wind speeds of almost 250 kms per hour, it ravaged through the land, clearing out everything in its path. Fields were left barren, trees uprooted like mere matchsticks, entire towns devastated. More than 10,000 people lost their lives.
Fast forward to two decades later. In 2020, bang in the middle of the Covid-19 pandemic, another cyclone—known as Amphan—speeds through the Bay of Bengal. It crashes into the land like Paradip did in 1999. Like before, many homes are destroyed and structures uprooted. But one thing is different: this time’s death toll is 98. That’s a 100 times lower than 1999’s casualties.
What made this difference possible? Simply put: better, timely and more accurate weather prediction.
Does this mean meteorologists have gotten better at weather forecasting? Not necessarily. Weather prediction has always been an unforgiving and difficult task. It’s one that Dr. Shafiqul Islam, a professor of Civil and Environmental Engineering and Water Diplomacy at Tufts University, and his team have been studying for years.
“I can predict exactly where the sun will be on the fourth of April at 11am,” Islam says. If you’re wondering how he’s able to do this, it’s because Professor Islam and team have developed certain basic mathematical methods to know how the planets are moving.
“That gives us a very strong certainty that things are extremely predictable,” he says. “I can tell you where the sun will be a hundred years from now, I don’t have to relearn astrology. But the India Meteorological Department, or even the National Institute in Boston, cannot tell me if it will rain on Friday.”
In simple words, Professor Islam is trying to explain why weather prediction is incredibly complex. There are some things you can predict reliably and many other things that you just can’t.
“The difference is that although these are based on fundamental mathematics and physics, the physics is different,” he goes on. “The physics for celestial bodies is predictable, it’s more or less linear. But the physics for weather is extremely non-linear. It depends on the initial conditions, it depends on the boundary conditions. As a result of a small error that you make in the initial condition, it will blow up in a few days.”
In fact, weather forecasting is as good as the initial data we receive. Once we get some variables—say temperature, pressure, or humidity—we make a prediction. Now, the interesting part is that over many decades, weather stations around the world have managed to improve the volume of data that they are able to gather.
“The physics for celestial bodies is predictable, it’s more or less linear. But the physics for weather is extremely non-linear.”
Today, organisations like the IMD can station ships deep in the ocean to receive information about water temperatures; they have satellites up in the sky to track clouds; there are buoys placed across the waters to get a sense of the ocean currents; there are doppler radars and anemometers for information about wind speeds. All of this helps a meteorologist condense disparate bits and pieces of data into a single mathematical equation.
And the result of this equation? It’s what we see in those one-line weather forecasts on news channel tickers at the bottom of our screens, and the predictions on our phone’s weather app.
Now, if there’s even a slight error in the data collected, our forecast can go haywire. That’s not all. Despite all the scientific progress we’ve made so far, there are still a million variables that affect the weather that we don’t even know of.
But that’s a story for another day. For now, let’s focus on the data that meteorologists can actually gather. At its very core, predicting the weather is fundamentally a data cleaning exercise. But a massive, massive one.
eather prediction has so many variables that it’s almost impossible for any human mind. Even a simple temperature reading requires tons of data to be processed. It is here that scientists and researchers are hoping that Artificial Intelligence can play a role. The hope is that AI can be used to sift through the data, do its little magic between its neural networks, and pop out a weather prediction accurate to the dot.
To achieve this, AI requires systems that have extremely advanced GPUs and microprocessors that can undertake the complex calculations that are required by machine learning algorithms.
OpenAI, a US-based for-profit AI research lab, found out in 2018 that the computational power required to train the largest AI models doubles approximately every three-and-a-half months. By the way, this is the same lab that created a robotic arm called Dactyl, that could successfully solve a Rubik’s Cube. But it needed more than a 1000 desktop computers and a dozen advanced graphic chips to run for several months to make that happen—and even then, the cube fell out of Dactyl’s hands 8 times out of ten.
Fortunately, India has made some progress in this area. And that’s by embracing supercomputers. India purchased its first supercomputer in 1988. It was called the CRAY XMP. It had a processing speed of 400 megaflops. This may seem minuscule in today’s world of petaflops. But back in the 1980s, it was revolutionary.
Manmeet Singh, scientist at the Indian Institute of Tropical Meteorology in Pune, says that today’s supercomputers are more powerful. “They have a much much higher disk storage capacity, their computational power has increased manifold,” Singh says. “Today we stand at par with international centres who use supercomputers for weather forecasting.”
The Indian Institute of Tropical Meteorology began as a wing of the IMD, but it’s now an autonomous institute under the Indian government’s Ministry of Earth Sciences. The sharpest minds in climate academia, oceanography and meteorology are groomed here. Its scientists and researchers are known to go all out with their experiments. Little surprise then, that India’s fastest ever supercomputer was created here in 2018.
Manmeet Singh has been with the IITM since 2013. He does extensive research on climate models, and in figuring out how to use AI and machine learning to improve traditional methods of weather forecasting like the Numerical Weather Prediction model, or the NWP.
“We have to understand that we live near the tropics, we are near the equator, where we get a larger proportion of sunlight as compared to people who live in the mid latitudes or the poles,” Singh explained. “So the energy in our system is higher, the turbulence is higher, we have a much more chaotic system.”
Scientists may be able to predict the onset of a storm way in advance. However, they will be able to tell you how severe it’s going to be only two or three days in advance. “Satellites have provided a very good opportunity for us in the last few years,” Singh says, “but we cannot go up and set up an instrument in the air and measure exactly when the rain droplet will form, when will the water vapour get converted to a rain droplet.”
That’s where the Numerical Weather Prediction model comes in. It boils weather forecasting down to not-so-simple mathematical equations. This helps forecasters make educated guesses about what the weather will look like, moving ahead. But the climate in the tropical region of the Earth is so chaotic. So, things change often and rapidly. With the assistance of big systems with huge computational power, this accuracy could improve. The IMD has already begun experimenting with improving the prediction model using AI.
But the sheer amount of data it takes to make accurate weather forecasts is so vast. We’re not quite there yet. We need more resources to forecast weather with the highest level of accuracy.
“Now the computations happen predominantly in a single GPU,” Manmeet Singh tells us, referring to a graphics processing unit. “It's very difficult as of now to use multiple GPUs, and combine the multiple GPUs, and train a single Artificial Intelligence model.”
“Think of this as two containers full of water, with a pipeline connecting the two. But the pipeline is very thin, or it is very small. So, the amount of time it takes to transfer data from one GPU to the other, that’s a big bottleneck in the present systems. We can use only a single GPU at a time.”
“It depends on the number of floating points operations we can do per second. A GPU has thousands, of course. It’s much faster to use TPUs (or tensor processing units) and others which are not yet available to us commercially. It eventually boils down to the hardware that we use.”
“We cannot go up and set up an instrument in the air and measure exactly when the rain droplet will form, when will the water vapour get converted to a rain droplet.”
“Now, this is the challenge posed by the sheer volume of data. Simultaneously, there is also the challenge of making predictions in a timely manner. You see, time is of the essence in situations like a cyclone. The earlier we’re able to evacuate people, the more lives we are able to save. And we can only evacuate people early if we’re able to come up with an accurate forecast well in advance.”
But according to Professor Islam, we’re in a bit of trouble here. “I am now making a very strong assertion that all these weather models are not going to be able to predict beyond seven days with accuracy,” he says.
Clearly, he’s not mincing words here. Remember the numerical model we spoke of earlier? That’s what Professor Islam is talking about. A quick recap: this model collects data and uses mathematical equations to make a prediction.
“It almost becomes like flipping a coin,” Professor Islam says. “The IMD is not to be blamed. They will be very good at predicting the weather three or four days in advance. Beyond that, it cannot be done because there is a fundamental mathematical limitation to these questions.”
“IMD can do another sensor, another satellite, they can maybe improve predictions from two days, to three days. But can they do ten days? There is no evidence. Right now there is no weather forecasting model on the globe. India to Europe to Australia, it doesn’t matter, it’s not there.”
t’s hard getting the answer right when your question changes every few hours.
Professor Islam and his team are in the thick of building an AI model that will allow them to predict a flood 15 days in advance. Like I said before, there’s a huge difference between predicting a flood five days in advance and 15 days in advance. India may have had to suffer $13 billion worth of damage during Amphan, but remember: thousands and thousands of lives were saved because of forewarnings.
“My objectives are very humble,” Professor Islam says. “I want to be better than traditional forecasting models. They’re doing four to five days, I want to be able to predict ten days so we can give communities enough time to move.”
“Are there patterns of events happening on the Indian Ocean today that will have an effect on the weather in Boston in 15 days? We want to understand that. Can these types of connections be established? These are difficult. We know there won’t be a prediction of exact values, but are there patterns which are predictable?”
The professor has a cautious approach to weather prediction, though. To explain, he quotes Edward Lorenz, the man who established the base theory for weather prediction back in the 1950s and 1960s.
“There is a very famous metaphor Lorenz uses: how a butterfly moving its wings in Beijing can create a thunderstorm in New York two weeks from now. He’s trying to explain that a very small change in initial condition can have a very large outcome in a faraway place,” Professor Islam says. “Because of the non-linearity of the equation. Now, I have lots of data coming from all kinds of sources, which is complementary to my model. What I am trying to get a sense of, really, is this: can I use AI to combine my fundamental equations and my data, to find out a pattern which is predictable for more than five to seven days? Here is where AI could be extremely powerful technically. Just a blind use of AI will not work.”
“We have an illusion that AI will solve all our problems if we can bring it to an angle, yes,” he adds. “I think it is extremely powerful, but we need to be cognizant of what it really can or cannot do.”
The weather might seem chaotic and non-linear to us, but AI might have the ability to recognise a pattern or a behaviour that we haven’t found the mathematical equation for yet. In essence, Professor Islam believes that AI works best when there are behavioural patterns. The neural networks that make up an AI model are dependent on the data, or history, that is fed into it. They learn from this data, interpret a pattern, and predict what could happen in the future based on this pattern.
“I give this example to my students,” the professor says, “Look, usually all of science and AI is based on this idea of driving with the rearview mirror. I don’t have an option, so I’m looking into my rearview mirror and driving. I will be fine as long as the road in the front exactly mirrors the rearview. If it doesn’t and I go into a turn? Disastrous accident.”
It’s why AI machines are exceptional at playing games like chess, where they can study thousands of previous games. But the same technology is yet to truly grasp art and culture, born out of the sheer creative force of the human mind.
It’s true that AI can only help us to a certain extent in confronting some of the most challenging aspects of weather prediction. But this isn’t the whole story. Here’s a different application of AI in weather forecasting: one that is not only creative, but also offers hope.
Anshu Sharma is the founder of SEEDS India, an organisation that works to provide relief for those affected by natural disasters. He is also a recipient of the Microsoft AI for Humanitarian Action grant.
Initially, Sharma’s work began as a simple response and reaction organisation, which landed up in disaster-hit areas to rebuild all that was lost in the aftermath of a climate event. But today, it has turned into something visionary.
Sharma also started with a cyclone: Fani, in 2019. While surveying the damage around him, seeing homes dismantled and buildings rattled, Sharma had an epiphany. He realised that the brunt of such disasters is often borne by the poorest communities. But with enough planning and research, could someone assess what property is at risk and find a way to mitigate it well before disaster strikes?
“It hit us that the damage that happened to the buildings in the poorer communities could have been anticipated very precisely at each building’s level,” Sharma explains. “We could have told in advance which roofs would fly away, and which walls would collapse, which not so. So that's something we’ve tried to bring into the AI model, so that it can be a predictive model.”
“What we do is, essentially, this: we use satellite imagery or drone imagery and through that, we identify buildings. We identify the material and technology of the building through their roofs as a proxy, and then we simulate a sort of a risk assessment of that particular building, right? We also take the neighbouring context into account. Water bodies, how high or low the land is, what kind of trees are around it, what other buildings are around it.”
The team at SEEDS and many others like it are more concerned about the micro-impact that weather events will have. Sharma is interested in the specifics of how long a building made from a certain material will be able to withstand the force of a cyclone. This is an advanced level of interpretation we’re talking about. It’s creative, important and life-saving as well.
“We add a layer below the weather layer, we add a layer ki kaun log hai”—who are the people there?—“what kind of buildings are there, what kind of infrastructure is there, what vulnerabilities they have,” he says. “Is it a fishing slum? Is it a high income group, multi storied apartment building? That’ll make a life-and-death difference.”
Sounds like such a simple idea, right? If we can predict the weather, surely we can anticipate its impact as well. It’s this kind of optimistic use of AI that could help save millions of lives in the future while keeping structural losses to a minimum.
ut we’re reckoning with perhaps the biggest anxiety humanity has faced in its collective future: climate change.
As depressing and worrying as it may be, climate change is here and it is a conversation we must have. The question is: what kind of a role can Artificial Intelligence play here?
In 2015, parts of India were swept by a severe heat wave. The earth was scorched dry, sucked of its water. The heat was relentless. A video of a man breaking an egg and frying it on the road in Odisha went viral, and became a humorous instance of how ridiculous the heat had gotten. Jokes are a way of coping with disaster for some, but this heat wave was nothing less than a catastrophe. 2500 deaths were recorded. Many hundreds more unrecorded.
Just last year, Delhi experienced yet another heat wave in July. Temperatures were a whopping seven degrees higher than average. Remember, researchers predicted an average increase of 1.5 degrees over the next five years. Seven degrees higher than normal is a temperature that our bodies are not equipped to deal with. A cyclone or an earthquake are physical events that cause tangible damage that can be dealt with at a certain level. But how does one deal with heat?
“Earlier, heat stroke used to be an outdoor problem, but with the kind of materials we use for construction now and the climate that is changing, indoor heat has become an issue.”
“Earlier, heat stroke used to be an outdoor problem, but with the kind of materials we use for construction now and the climate that is changing, indoor heat has become an issue,” says Anshu Sharma. “It’s an issue in two ways. We’re first concerned about the poor who don’t have the ability to turn on air conditioners. They suffer in this heat: you will be surprised to know that a slum area in a city is about 5 to 7 degrees warmer than other areas.”
The lack of greenery and the poor building material used to build slum homes contribute to the severe increase in indoor temperatures. So if outdoor temperatures are 35-38 degrees Celsius, it could go up to 43-45 degrees indoors.
“And 45 indoors is a nightmare,” Sharma says. “Din mein kaun hota hai andar?”––who’s indoors in the daytime? “It’s old people, young children, their mothers, people with disabilities. So, we decided to run the model and come up with clusters of buildings that we knew would heat up very badly and have a severe indoor heat environment.”
Immediately, they’d reach out to inform these clusters about the days to come. “And we have a set of solutions for what they can do, right down to if there is an emergency,” Sharma says. “Also, if someone falls ill, what are the steps to be taken? And as you can imagine, the huge difference is that right now, the Met Department gives a warning for the whole city. But we are able to calibrate it. We’re able to find out who’s going to be worst hit.”
So, while things get more and more dire, there is still some hope. And just like SEEDS has recalibrated and adjusted their vision to meet the challenges of climate change, so will other applications of Artificial Intelligence, believes Professor Manmeet Singh.
“There are lots and lots of applications, wherein AI can come into play for the basic understanding of climate change, understanding the science, mitigation impacts, and so on. This includes, for example, enabling low carbon electricity or reducing current system impacts,” Professor Singh says. “Uniting data machine learning and climate science, and forecasting extreme events will help governments, cities, and organisations like ours have information beforehand and plan ahead. Artificial Intelligence is the way to go and as I said about climate prediction, we need to know what will happen in the future to be prepared.”
Professor Islam has a more nuanced view.
“This idea that AI can help in predicting climate change? No,” he says outright. “AI can create simulations based on what we have done before. What AI can do is come up with some adaptive strategies, based on scenarios. So it can show you ‘Given this scenario, say, this can happen in 10-20 years; this is what happened in Brazil in a similar scenario and this is the way you can adapt.’”
We may not have all the answers just yet. But the fact remains that Artificial Intelligence is going to alter how we see the world, how we react to things and how we understand them. The history of the planet is filled with sordid stories of cities and civilizations being wiped out because of their inability to deal with natural disasters. But we may perhaps be the first ever human generation to be at least somewhat prepared for any kind of natural disaster.
It’s a start. How far will we go? Only time will tell.
Prthvir Solanki is a Goa-based writer. He otherwise works on short-form and long-form scripts for the screen. He also is a SouthAsia Speaks 2022 fellow, working on a book of short stories that he expects you to purchase the moment it hits the shelves of your nearest bookstore.